Monday, September 3rd , 2012
Yiannis Demiris, Imperial College London.
Title: Bidirectional Assistance in Human Robot Interaction
Abstract: As robots and humans increasingly co-exist in home, medical, industrial and entertainment environments, mechanisms for mutual assistance become increasingly crucial. Novel task knowledge can be demonstrated by the human, and learned by robots through observation and imitation, while robots can also build adaptive user models and modify their level of assistance depending on the short and long term inference of the human's needs.
In this talk, I will review our research on bidirectional assistance between humans and robots. I will first review statistical, one-shot, and grammatical approaches to learning by imitation in humanoid robots, followed by how robots can modify their level of assistance to adapt to changing user needs, giving examples from assistive robotic wheelchairs for children and adults, and adaptive robotic dance tutors for hospitalised children.
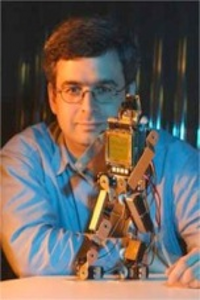
Bio: Yiannis Demiris is a Reader in intelligent and assistive robotics at the department of Electrical and Electronic Engineering at Imperial College London, where he heads the Personal Robotics laboratory. He does research on human-robot interaction, humanoid & assistive robotics, and multi-robot systems, with a particular emphasis on the mechanisms of development and learning.
Yiannis Demirs received his PhD in Intelligent Robotics from the Department of Artificial Intelligence of the University of Edinburgh in 1999, and joined the faculty of Imperial College in 2001. He has organized several international conferences, including the IEEE conference on Development and Learning (ICDL-2007) as chair and the ACM/IEEE conference on Human Robot Interaction (HRI-2008) as program chair. He has edited three books on robot learning and guest edited special journal issues of the IEEE Transactions on Systems, Man and Cybernetics (Part-B) on Robot Learning by Demonstration and Imitation, and the journal of Adaptive Behaviour on developmental robotics. He has received fellowships from the European Science Foundation and AIST in Japan, and is a member of EPSRC College, and a senior member of the IEEE. He is participating in two European FP7 projects, ALIZ-E (Adaptive Strategies for Sustainable Long-Term interaction) and EFAA (Experimental Functional Android Assistant), while collaborating in industrial research projects with BAE Systems, and IBM. In 2012 he received the Rector's Award for Excellence in Teaching, and the Faculty of Engineering "Excellence in Engineering Education" Award.
ProgrammeTuesday, September 4th , 2012
Jan Peters, Technische Universitaet Darmstadt.
Title: Towards Motor Skill Learning for Robotics
Abstract: Autonomous robots that can assist humans in situations of daily life have been a long standing vision of robotics, artificial intelligence, and cognitive sciences. A first step towards this goal is to create robots that can learn tasks triggered by environmental context or higher level instruction. However, learning techniques have yet to live up to this promise as only few methods manage to scale to highdimensional manipulator or humanoid robots. In this talk, we investigate a general framework suitable for learning motor skills in robotics which is based on the principles behind many analytical robotics approaches. It involves generating a representation of motor skills by parameterized motor primitive policies acting as building blocks of movement generation, and a learned task execution module that transforms these movements into motor commands. We discuss learning on three different levels of abstraction, i.e., learning for accurate control is needed to execute, learning of motor primitives is needed to acquire simple movements, and learning of the task-dependent "hyperparameters" of these motor primitives allows learning complex tasks. We discuss task-appropriate learning approaches for imitation learning, model learning and reinforcement learning for robots with many degrees of freedom. Empirical evaluations on a several robot systems illustrate the effectiveness and applicability to learning control on an anthropomorphic robot arm.
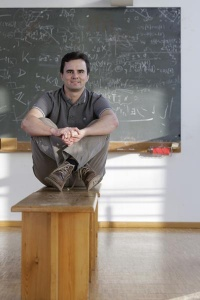
Bio: Jan Peters is a full professor at the Technische Universitaet Darmstadt and a senior research scientist at the Max Planck Institute for Intelligent Systems (MPI -IS) heading the interdepartmental robot learning group. Until 2011, he was was senior research scientist at the Dept. for Empirical Inference and Machine Learning of the Max Planck Institute for Biological Cybernetics (MPI-KYB) in Tuebingen, Germany. He graduated from University of Southern California (USC) with a Ph.D. in Computer Science. He has completed masters degrees in Electrical Engineering (Dipl.-Ing./TU Muenchen), Informatics (Dipl-Inform./FernUni Hagen), Computer Science (M.Sc./USC) and Mechanical Engineering (M.Sc./USC). Jan Peters has been a visiting researcher at the Department of Robotics at the German Aerospace Research Center (DLR) in Oberpfaffenhofen, Germany, at Siemens Advanced Engineering (SAE) in Singapore, at the National University of Singapore (NUS), and at the Department of Humanoid Robotics and Computational Neuroscience at the Advanded Telecommunication Research (ATR) Center in Kyoto, Japan. His research interests include robotics, nonlinear control, machine learning, reinforcement learning, and motor skill learning.
Programme
This work is licensed under a Creative Commons Attribution 3.0 License.